Who should we spend more time with?
When investors underwrite seed-stage companies, the new company’s team and product, and even market, might have not yet materialized. Even with so much uncertainty, investors still develop confidence, but how? If you asked many seed investors, you would get just as many distinct answers, but the most concrete (and possibly only) unifying themes would be special relationships with talented entrepreneurs and a differentiated deal network.
At Tribe, we’re always searching for businesses and investment partners who are additive complements to our core strategy of recognizing and amplifying product-market fit. We’ve elaborated our framework for measuring product-market fit in a previous post, and here we will share some of how we think about investor partnerships.
The earlier in a company’s lifecycle, the more relationships matter, and at the seed stage, relationships are everything. Of the thousands of possible seed investing partners, which relationships should we develop? How should we spend our time? These questions drove us to develop a systematic strategy to help us spend our time smarter, within and beyond our immediate line of sight.
Why and how we think about track records
We believe VC is a high skill asset class because the best VCs are a lot better than the average, and because VCs that invest well in one fund tend to invest well in subsequent funds. If the motion was pure luck, winners in one round would not tend to be winners in subsequent rounds. To us, this means track records matter.
But “good” can be extremely subjective, and in fact different LPs, entrepreneurs and VCs will look for different characteristics when partnering to solve their particular business problems. One entrepreneur might want fast funding, another might want a well connected partner for follow-on rounds. One LP might want broad exposure and another might want concentrated moonshots. Further, investors with great processes don’t always end up with good returns, and investors with bad processes sometimes have great returns. This leads us to our core realization:
We need to be able to measure proxies for good investment processes across a spectrum of investing styles
We settled on three key factors to capture seed investing track records:
- How strong are the returns of an investor’s seed portfolio?
- How Active is an Investor at the Seed Stage?
- How has subsequent fundraising progressed?
There is no single best investor — every has different degrees of different types of strengths, and our model needs to notice when an investor is showing any signals of extraordinary skill.
Finding outliers
To capture when investors are doing something special, we need to (a) measure indicators of good investment processes and then (b) combine the indicators to produce a gauge of overall track records. Well-designed logic in this way captures a multitude of investing styles and processes and flags when something extraordinary is happening.
Without getting too into the statistics of our approach, we model the joint probability distributions of a variety of investment metrics and then combine them to form our final indicator.
We found that binary indicators — “hit/miss” processes, like whether or not a Seed-stage company reaches Series A — are great building block metrics. The variance on unbounded metrics, like realized returns from an IPO, can be astronomical — measuring hits and misses is comparatively resilient. In a way, because VC outcomes tend to be binary, this representation is natural.
Some example metrics are …
- Did the company raise a Series A within 3 years of their Seed?
- Did the company raise $25M within 5 years of their Seed?
- Did the company get acquired beyond liquidation preference within 6 years?
Notice that these metrics require fixed windows, which introduces lag and shrinks the available sample size, but reducing bias is worth it. We can then assemble many metrics into a holistic model:
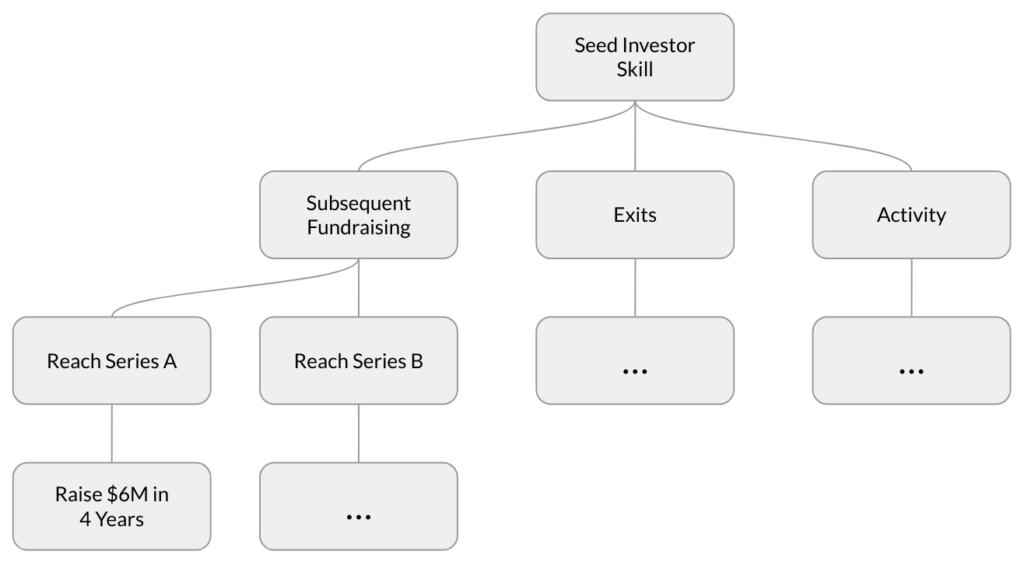
The concept of Abstraction is key here, where we distill the essence of multiple constituent metrics. Each base measurement, like “Raise $6M in 4 years,” captures granular details which are subsequently combined, simplified, and synthesized into higher-level concepts.
Looking forward…
We are in the early days of putting these models to work, and have broadly found 5 useful seed investing styles outlined below (and in more depth in the FAQ):
Strength | Examples | Appeals to… |
Strong exits Investments IPO or return beyond liquidation preference | Accel, KPCB, Elad Gil | Entrepreneurs seeking blue-chip board, LPs |
Reach Growth Stage Investments tend to reach Series B+ | Sequoia, Battery, Lee Linden | Investors who want low loss ratiosSeries A investors |
Early Signs of Future Success Investments tend to reach Series A+ | Madrona, Forerunner, Chip Hazard | High-burn early products, Series B investors |
Very Active High counts + well rounded | First Round, Khosla, Sam Altman | Fast capital, deal flow |
Multiple Signals Similarly strong in 2+ categories | Initial, Samsung, Eric Paley | Varies |
If we want to increase deal flow, we reach out to the top “Very Active” investors, which tend to see many deals while also being outstanding generalists. If we’d like to have a more concentrated portfolio and be more active in our portfolio, we’ll spend more time with top “Reach Growth Stage” strategists.
We’ve also made progress exploring the investing network to develop Coincident Coinvestor models. Since Track Record models capture decisions made many years ago, we believe coincident models can unlock the ability to capture recent developments (changes in strategy or quality), diamonds in the rough (hidden and unknown investors with a great network) and rising stars (brand new investors on rocket ships).
At Tribe we’re focused on partnering with the best entrepreneurs and investors, and we laid out the principles and framework for one way we level-up our strategic time spend. We hope this serves as inspiration for your own innovations and would love to hear any questions, feedback or ideas — feel free to reach out to hello@tribecap.co.
FAQ
Why did you look at a variety of factors versus focusing deeply on returns?
In baseball, each game is decided by total runs scored. Suppose there was a player that hits twice as many doubles as the average player, but is rarely the one crossing the plate and scoring a run. Would you not want that player on your team just because he doesn’t have many runs? In reality, that player is doing something important very well and could be a great addition to the right team.
There’s not one type of good investor, just like there’s not one best type of baseball player. Different investors have different strengths which are strategic complements for different types of investors, entrepreneurs, and LPs. For example, an entrepreneur with a capital-intensive business might be looking for a rainmaker, an LP with 10+ year horizon allocation planning might prioritize returns, and an early-stage investor might be looking for partners with high activity and deal flow. We acknowledge this and account for it in our analysis.
Not to mention returns are subject to incredible variance. Unpredictable factors matter a lot in seed investing, and low MOIC might not even indicate worse-than-average investing, since repeating the same process could result in a positive outcome. Process matters, and the leading indicators under the hood, like intermediate milestones, are illuminating.
Who is counted as an investor?
There are over 25,000 institutions and individuals in the total available set of seed investors in the US at the time of writing. Who is counted in part depends on an eligibility criteria, often set by:
- The time window where we consider an investment relevant, such as all investments between 2010 and 2015
- The geographic scope
- A minimum seed count to be able to develop reasonable confidence
- What is counted as a seed investment
The tradeoffs we consider when setting the bounds of the “time window” are how the macro environment has shifted, how relevant older decisions are, the fact that more investments give us more information (bigger window is better) and how much time we’d need for milestones to unfold. Note that in the construction of a particular metric we need to cut off all outcomes by the same window — Seed to A in 4 years means the latest we can look is 4 years in the past, and if we observe a seed investment in 2014, we have to cut off subsequent milestones to 2018, even if the company ends up raising its A in 2019.
We typically use the USA for geographic scope or our purposes, but this can easily be expanded to other geographies. In fact, we can use the scoping in broader ways than just Geography, such as particular verticals (“Fintech” or “Agriculture”). In practice, “minimum seed count to develop confidence” is a computational simplification and our Bayesian method otherwise handles this.
For “what is counted as a seed investment”, an example is to define a seed investment as any amount of participation in a round identified as Seed, Pre Seed, Angel, or Convertible Note or any investment when, after the investment was made, the company’s cumulative funding was under $4M. You can imagine ways to define this that vary over time to handle heat and inflation, but simpler is better. Individuals track records include their angel investing in addition to any activity in which they were attributed to the deal on behalf of an organization.
As an example, of the 25,000 available, if we set a minimum seed investment count of 5, and required at least 4 years of history and included all investors back to 2008, we would have a set of 2,100 individuals and organizations. We included investors with 5 or more seed investments in the USA between June 2008 and June 2015.
Are investments 5 or more years ago ancient history?
Five years is a long time, and consumer demand and the strength of businesses certainly changes over such a timeframe. But in a way, these changes necessary in order to gauge the success of venture capital investments. VC is a long, slow game where the true value is only unlocked by such secular shifts.
It is not possible, absent knowing the intricate details of an investor’s decision process, to gauge the quality of a seed investor’s decisions without waiting to see how things turn out. This is compounded by the fact that we need many examples to handle the high outcome variance. Even in public market investing with dramatically shorter horizons decades are necessary to truly discern investor skill, and the reality is time and patience are the cost of doing business when assessing track records. In many ways, 5 years is actually not such a long time horizon after all!
Fortunately, while the world changes, good VCs tend perform well consistently, and tend to remain in the game for a long time. So discerning who made good decisions 5 years ago is in fact a good indicator of who is making good indications today.
The main challenge with this approach is it precludes us from seeing the most valuable signal: up-and-coming investors who have not yet made names for themselves. As mentioned above, we have developed frameworks for assessing coincident investor scores, which we hope to share soon.
What do the scores mean? How should we compare investors of different ranks?
To understand the scores let’s first remind ourselves why we looked at seed investors in the first place: to know who (among the thousands) to spend time with and develop productive partnerships. We can’t spend time with every good potential partner, let alone know who they are, but perhaps we can at least spot positive outliers with high confidence.
But what does “good” mean in this context? In reality, there are many different types of good investors, and different types of leading indicators of success. To reiterate, our core factors are:
- Intermediate fundraising milestones (Series A/B/… and dollar milestones)
- How often seed investments successfully exit (i.e. return meaningfully above liquidation preference or IPO)
- Level of investment activity
Using these inputs we construct statistical models of each investor, and based on their track records we can develop certainty around outlier statuses.
Hence there is no best investor, some are better at some things than others, and the model is designed to notice when an investor is showing signals of extraordinary skill. Our scores, and the resulting ranking, captures how confident we are that each investor has done (and is doing) something special. The higher ranked, the more confident we are.
What are the types of strengths that differentiate the best seed investors from the average?
Loosely, we found 5 categories can describe the underlying drivers behind why investors can be considered top-tier:
- Strong Exits. Top investors with strong exits show weaker early signals but have had outlier exit events, such as high-priced acquisitions and IPOs. These investors often have excellent track records but can be funds that “graduate” up; who succeed as seed investors, raise larger funds and move up-stage. These investors might alternatively be particularly skilled at identifying frontier opportunities and might be less involved with their portfolio in the short term, and could be of interest to LPs, low-burn entrepreneurs, or those interested in learning about new markets.
- Reach Growth Stage. This means the investor had particularly notable rates of seed investments that go on to raise tens of millions of dollars, reaching and surpassing Series B. These investors could be good partners for entrepreneurs whose business models have a wedge that they’d pivot into adjacent markets or move up-market. These investors are also good partners for Series A investors because they drive success before and after Series A investments.
- Early Signs of Future Success. Seed investors that show early signs of future success have an outstanding ability to identify entrepreneurs that advance products beyond MVP and make it to market. These investors tend to have tailwinds for future fund performance in 4-6 years. They are in a sense “rainmakers” — while the typical seed investor might have a 30% rate of seed investments raising a Series A, these investors would have rates as high as 80%. Entrepreneurs that need the next level of capital to test a product, such as a consumer social media company that needs a critical mass to test their idea, could benefit from these partners. Growth-stage investors could also be interested in these seed investors to learn what the Series B pipeline might look in 4 years as they map strategic themes.
- Very Active. The more active an investor is, the more confident our picture is of their investing ability and style. Top investors who are very active tend to have good but not unbelievable individual drivers — on the contrary, they have “pretty good” performance all-around, all while being very active. Being “top quartile” in any single attribute isn’t particularly remarkable, but being “top quartile” in every underlying indicator is rare. This, combined with high activity, allows us to confidently see rare breadth of ability executed consistently. These investors tend to be good generalists and are attractive to those looking for quick funding or deal flow.
- Multiple Signals. Investors with multiple signals are similarly strong in two or more of the attributes above. That multiple performance metrics are strong and no single factor clearly exceeds the rest. These investors come in many strategic varieties, and could fit tailored strategic interests. Generally, digging deeper into the strategies of investors of this category is important to understanding potential synergies.
Can you click down into how you account financial returns and changes in valuations?
Financial returns definitely factor into our analysis, but we intentionally do not deeply index on “major hits”. This is because (a) returns themselves have high variation because of the nature of venture, and (b) past returns do not imply future success, and we aim for signals that indicate a high probability of success in the future (i.e. leading indicators). In essence this was the challenge and, in our opinion, the innovation: to gracefully handle these high-variance outcomes to produce something sensible and reliable.
“Successful exits” was probably the most statistically complex indicator because these events are so rare. Without getting into the details, we account for liquidation preference and handle the high rate of unknown valuations during an exit. That is, a successful exit is one that produces returns on the seed check, which tends to be binary.
The model also handles concepts of “heat” and “inflation”. For example, higher valuations could be due to a capital availability, and they could also be due to lower perceived risk in the asset class after more transformational tech companies emerged. It’s probably a bit of both and we didn’t want to be too assertive here. That’s why we broadened our metrics across these two possibilities by combining valuation sensitive metrics (i.e. dollar milestones) and valuation insensitive metrics (Series A/B/etc).
What are some limitations with the model and data sources?
It is important to separate the methodology from the model. Over time, we expect our data to augment or improve while retaining the fundamental concepts and philosophy behind our model outlined above.
In its current iteration, many new investors who might be very skilled are out of scope definitionally because their track records are nascent. Further, the model is lagged, as we are analyzing decisions made 4 to 10 years in the past. We are experimenting with models that analyze the coinvestment network to address the above. That said, even with complete data and a perfect model, randomness in venture outcomes will always produce false positives (investors who appear good but got lucky). Further, our model directly represents our business and domain expertise in the form of a logic tree. While this was intentional in the design, it means that we are limited by our understanding of the ecosystem.
On the side of our input data, we are limited in terms of data completeness and quality. We lack cap tables and accurate valuations. Participating with a $25k seed check for 0.5% of a company is a very different decision than leading the round with a $1M check for 20% of a company. Our data is also subject to many biases such as lag between funding and announcement, selection bias from when companies announce or associate with a funding round, and so on. Acknowledging, understanding and controlling for biases is crucial. There are also other relevant data, like investor talent and experience, web presence or social media activity, or other alternative data that may all be useful but are not yet incorporated into our model.
Can you elaborate on why you focused on investors when developing a quantitative approach?
We are part of a broad ecosystem of partners at all stages. Within that ecosystem, we focus on recognizing and amplifying product-market fit. As such, we have a particular affinity for partnering with seed investors for their ability to identify nexuses of entrepreneurial talent, and our ability to work with those entrepreneurs through inflection points in achieving product-market fit. It is important for us to know which seed investor relationships we should develop without limiting ourselves to our immediate networks. As it turns out, seed investing data, while still limited, is the some of most abundant in VC because there are just so many more seed rounds than there are at the later stages. Individual companies on the other hand, especially at the seed stage, have very limited or noisy data. At that stage other very important soft (“is this a strong team?”) or ambiguous (“is the market large?”) indicators are quite challenging to quantify.
How could the results be backtested?
Backtesting is a common and useful method for seeing how a strategy or model would have done after the fact. Backtesting helps find strengths and weaknesses in a strategy and allows us to not only iterate but also develop confidence. To do this, one typically needs to eliminate foreknowledge (to have a proper out-of-sample test) and account for realistic constraints, costs, and other factors which would be present under a realistic execution scenario. The discipline of backtesting in Venture is extremely nascent, and there is not yet a set of best practices or known playbook.
But in our opinion the core question to how one would backtest is “how would the outputs of the model be used?” Since there is no liquid or easily / widely tradable market price in VC, it is often helpful to reframe the question to be “what would I have liked to know?” This latter question is quantifiable via metrics — for example, if we wanted maximum coverage, we would have liked to know of as much deal flow as possible. If we were an LP looking for returns, we would have liked to know our model’s confidence of forward returns. Note that both the investment or fundraising objective and process dictate the design of a backtest.
Another important consideration is how one constructs a baseline to benchmark a model. One way is after the construction of a backtesting metric to compare many models and choose the one that one believes performs best (accounting for basic practices like handling bias, overfitting, etc), or to compare against naive models like a randomized investor ranking. To determine if model performance is statistically significant, we can bootstrap many versions of the model and baselines to bound the simulated metrics ex-post.